Solving LLM Adoption Problems
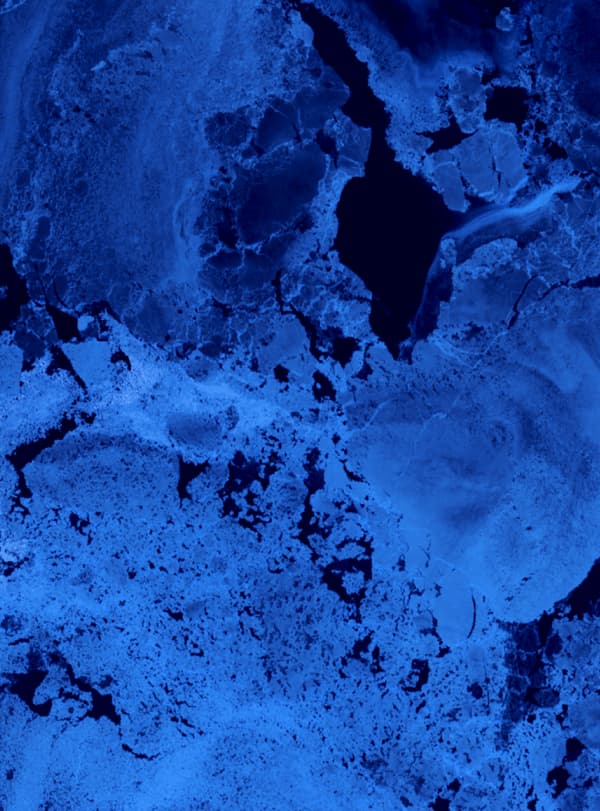
Adopting Large Language Models Safely and Securely at scale requires overcoming challenges of alignment, security, documentation, and trustworthiness.
We offer a robust suite of services designed to address these issues. Advai’s technology enables you to thoroughly test, align, and monitor these advanced systems.
We provide Testing, Evaluation, and Red Teaming services, developed from four years of research and our work with the most stringent of UK Government, Defence and Commercial partners.
Below, we'll zoom in on a few functions you can expect from Advai's Robustness Platform.
This is a version of Advai Insight customised for LLMs.
Our alignment framework securely interfaces with your on-premise or cloud hosted environment. We can run any LLM model through our library of 1st-party tests to benchmark performance, de-risk and secure the outputs of generative AI.
You can connect all your AI models so their health and performance, and the state of their risk and compliance markers, can all be viewed in one place.
The library shows broad information. The user can click into any specific use-case to see more granular information.
The dashboard is designed to bridge comprehension gaps and provide the right information to the right people.
At the top right of the image, you can see that a user can filter LLM testing information suited to their function. This selection changes the metrics shown.
View reports and track your compliance vitals, such as privacy and bias scores.
Metrics are customised to your industry.
For example, to the right, the Data Privacy and Protection score is 50%, indicating significant room for improvement in how personal data is protected.
Displays various cybersecurity vulnerabilities and the corresponding assessment scores, indicating the level of risk or the degree to which each area is secured.
For example, to the right, the Insecure Output Handling score shows 70%, indicating a moderate level of security concerning how the system outputs data, referring data being intercepted or misused.
The first step to LLM evaluation and control is to define what Assurance looks like for your organisation.
We work with you closely to define the specific governance requirements relevant to the AI system.
This includes identifying associated risks and outlining a set of controls that would enable you to effectively manage them.
This foundational stage sets the groundwork for secure and compliant AI use, with assurance mechanisms that prevent operational (i.e. misuse) and security (i.e. attacks) threats.
An AI governance framework is only as effective as its implementation and the clarity of human accountability in the AI ecosystem. Clear management understanding, operational guidelines, and user education fortifies the trustworthiness and reliability in your AI systems.
This step engages key stakeholders in understanding, contributing to, and aligning core organisational responsibilities with any AI implementation strategy, building AI literacy, responsibility and capability among relevant employees.
Determining vulnerabilities of large language models involves the crucial step of practical testing and evaluation. We draw on our extensive library of internally developed next-generation AI testing procedures. These procedures are designed to rigorously challenge AI systems and expose any potential weaknesses that could compromise performance or security.
This customised selection of red-team and adversarial tests are then mapped against your organisational objectives, risk frameworks and compliance requirements. This stage delivers clear technical metrics about how the AI system(s) perform under various challenging scenarios.
This fourth step is to implement a robust system of dashboarding and control measures. This maps technical metrics against the pertinent governance criteria, on a per-model basis.
Ongoing oversight is important because the reliability of language models, especially those subject to 3rd party updates, can change over time. This includes tracking LLM performance against the accuracy and reliability criteria determined in prior stages. Specific risks identified such as data privacy and bias need continuous monitoring against evolving standards.
Additionally, visual dashboards enable non-technical senior management to oversee LLM performance against risks and compliance, aligning this information with broader strategies for responsible AI use.