AI powered tests for ID verification systems.
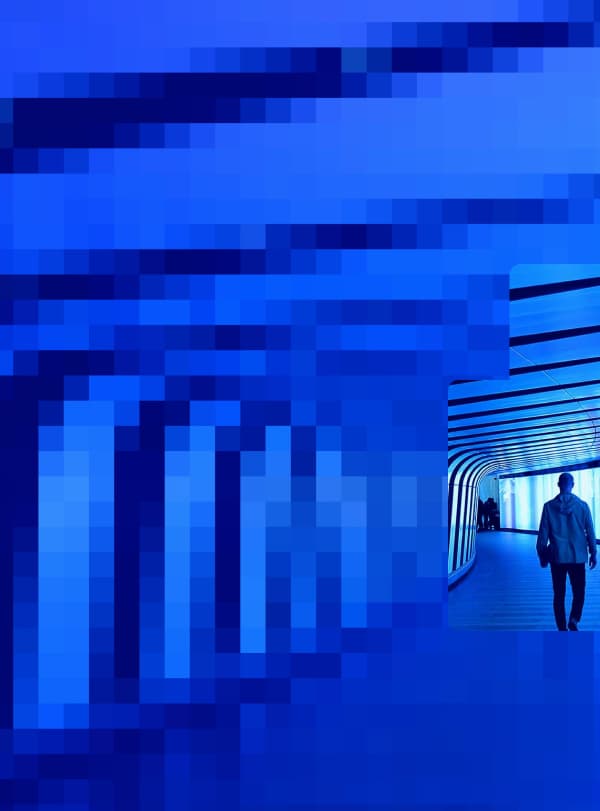
AI is core to online identity verification. As the complexity of verification tools have advanced, so too have the methods to deceive them.
Advanced machine learning claims are difficult to assess.
Advai can cross-evaluate different providers against the vulnerabilities most important to your organisation.
With an increased resilience to online threats, adversarial activity and fraud, have confidence when deploying your identification system.
Adversarial perturbations can be created to fool a system into thinking one person is another, as seen with Mark and Johnny here.
These perturbations can be invisible to the human eye. Therefore, human control checks miss these attacks.
Reduce your risk of fraud by measuring and improving the robustness of your verification provider's AI.
Quantified robustness will increase your confidence in deployment.
We can work with your vendor to suggest ways to strengthen their systems, specific to your risk appetite and unique industry needs.
Reduce the exclusion of legitimate users.
The real world comes with varying lighting conditions, device types, and potential biases in the training data (such as under represented genders or ethnicities, or even features like beards and glasses).
You can't test on regular samples because these are tests on the equivalent data models were trained on. We've developed methods to perturb sample images, massively increasing training data and improving real world resilience.
Comparative benchmarking helps you find a verification provider for optimal compliance and risk reduction.
Enhance your cost benefit analysis in vendor selection, enabling you to select a partner that matches your risk appetite.
We produce new information that helps you balance speed and accuracy, alongside resilience to adversarial activity.
Inhuman intelligence make inhuman errors.
Evaluate the performance claims with tests built to verify AI models, originally design to assure vision systems for the UK Ministry of Defence.
New strengths provided by AI systems also means new weaknesses. Deep knowledge from adversarial AI research pinpoints these vulnerabilities and strengthens systems against advanced AI powered attacks.
Discern between indistinguishable claims about accuracy and security.
Cut through the noise of marketing claims.
Reduce risks associated with uninformed decisions.
Save time and resources in vendor selection.